Humans in automated decision-making under the GDPR and AI Act
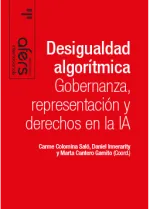
Anna Levitina, abogada especializada en tecnología, protección y privacidad de datos, y sistemas de toma de decisión automatizada (ADS); investigadora predoctoral, Universitat Autònoma de Barcelona (UAB). 1592113@uab.cat. ORCID: https://orcid.org/0009-0004-9855-5334
Human oversight is a fundamental safeguard against inappropriate judgments made by machines about people who are the targets of these decisions. Although the General Data Protection Regulation (GDPR) and the AI Act address human oversight to some extent, they fall short in addressing qualitative aspects and the integration of human overseers into governance frameworks. This paper examines the legal requirements for human oversight, investigating how these intersect with the accountability obligations of the automated decision-making (ADM) deployers and individual rights. It argues for a more comprehensive approach that not only includes human oversight, but also a continuous and rigorous assessment of the effectiveness of human control. Without that, human oversight may fail to protect adequately and could even worsen the impact on individuals affected by ADM.
As artificial intelligence (AI) continues to permeate our lives, the question of how automated decision-making systems (ADMSs), including AI systems, are governed becomes increasingly critical. The concept of human intervention governance, which involves maintaining human oversight and control over AI systems, is a key aspect of this discussion (Lazcoz and De Hert, 2022). In the European Union, the General Data Protection Regulation (GDPR) established a foundational framework for human involvement in ADM, introducing a meaningfulness qualifier that mandates substantive human participation in these processes.
Recognising the need for more comprehensive human oversight where high-risk AI systems are employed, the AI Act further mandates that overseers possess the necessary competence, training, and authority, and that their level of AI literacy be ensured. Despite these advancements, the GDPR and AI Act fall short in addressing qualitative aspects of human oversight and integrating human overseers into governance models.
This work contends that mandatory human governance requires further elaboration. It first examines the legal requirements for human oversight, analysing how they intertwine with ADM deployers’ accountability obligations and the protections afforded to affected individuals. It then explores how human overseers can be effectively integrated into ADM deployers’ governance models, where overseers are viewed as integral elements of compound decision-making processes that affect individuals protected by the law, rather than as standalone stakeholders.
Building upon the framework proposed by Lazcoz and De Hert, which argues that human intervention must be governed within governance mechanisms, and acknowledging the recent contribution by the Spanish Data Protection Agency (AEPD, 2024), which emphasises that assessing the degree of intervention must account for human overseers’ qualifications and diligence, this work asserts that organisational measures and impact assessment tools should not only address the presentation and meaningfulness of human intervention but also encompass criteria for the competence, knowledge, and moral character of human intervenors.
This work advocates for a holistic perspective on human-automated decision-making (HADM), treating both algorithmic and human agents as integral components of HADM. Effective governance of HADM as a whole is essential for ensuring that human-subordinated ADM systems serve the best interests of individuals and civil society.
Automated decision-making (ADM) and humans
In recent years, automated decision-making (ADM), the process of making decisions exclusively by automated means without direct human intervention, has reshaped traditional decision-making, thanks to advantages such as rapid data processing, scalability, and reduced human error. ADM systems (ADMSs) analyse vast amounts of data, detect patterns, and generate outcomes, including predictions and decisions, and as such they are valuable tools in numerous sectors, including finance, healthcare, and criminal justice.
The risks associated with the technologies that underpin ADM – particularly artificial intelligence (AI) capable of replacing human actors – have prompted its legislative regulation. The General Data Protection Regulation (GDPR), which emerged as a technology-neutral regulation, instituted a broad prohibition of ADM, and introduced additional safeguards for data subjects within the ADM domain. Recently, this regulatory framework has been supplemented by the AI Act (AIA) which, while aligning with the GDPR, focuses specifically on AI systems (AISs) and their associated risks.
ADMSs learn from historical data and may contain inherent biases which, if not adequately addressed, can lead to discriminatory outcomes, perpetuating social inequalities and impacting vulnerable populations disproportionately (Almada, 2019; Rovatsos et al., 2019). An example of this is the UK Home Office’s ADMS for visa applications, which was suspended following accusations of ‘entrenched racism’ due to its reliance on nationality as a risk factor in assessing applicants.
Furthermore, many advanced algorithms operate as ‘black boxes’, making it problematic to understand their decision-making processes (Schmidt et al., 2020). This lack of transparency and explainability raises questions of accountability and prevents individuals from challenging automated decisions, thereby limiting their control over important life-changing outcomes (Edwards and Veale, 2018; Roig, 2020). Constrained traceability and auditing limits in ADMSs obstruct the identification and rectification of errors, while also hindering regulatory compliance assessments and timely corrective actions (Berger et al., 2021; Berger et al., 2023). A case in point is the Netherlands’ SyRI system, used for detecting social welfare fraud and discontinued after six years of operation due to discriminatory practices based on income and ethnicity.
From a broader perspective, ADM poses threats to fundamental human rights, including data protection, privacy, human dignity, human autonomy, non-discrimination, and good administration. ADMSs may overlook the nuances of individual circumstances, reducing individuals to mere data points in algorithms, or impact personal autonomy, especially when automated decisions are binding and have far-reaching consequences. If deployed in public sectors, ADM may compromise public administration, depriving individuals of fair, justified, and contestable public decisions (Misuraca et al., 2020).
Accountability in ADM is central yet complex. In the case of non-compliance with substantive and procedural rules or standards, the responsibility for actions (or omissions) and their consequences should be assigned, and effective redress should be available for the affected individuals. However, there are concerns about whether existing accountability mechanisms are adequate, given the involvement of multiple parties in the development, deployment, and use of ADMSs, as well as their complex nature, learning capabilities, and unpredictability (Wieringa, 2023; Wagner, 2019).
Recent research underscores the need for a clear understanding of what it entails in terms of answerability, authority recognition, and limitation of power (Novelli et al., 2024). Human oversight is frequently cited as a primary mechanism for ensuring that ADMSs operate within the bounds of law and respect fundamental rights. However, growing concerns suggest that human oversight may not suffice to mitigate the risks associated with ADMSs, highlighting the need for clearly assigned roles and responsibilities, and for including affected individuals and the broader society in the oversight methodology (Kyriakou and Otterbacher, 2023; Green, 2022; Koulu, 2020).
The GDPR: data subjects and data controllers
Automated decision-making (ADM) and human intervention under the GDPR
At the heart of the ADM regulatory framework sits Article 22 of the GDPR. According to Article 22(1), ADM encompasses decisions based solely on automated processing, including profiling (the automated analysis of personal data to make predictions or decisions about individuals), that produce legal effects or similarly significantly affect individuals.
While constructed as a data subject right, Article 22(1) operates as a general prohibition of ADM, stipulating that ADMSs should involve a human review before the final decision is made. This provision is subject to the derogations detailed in Article 22(2) that permit ADM without ex ante human intervention either for contractual purposes, with the data subject’s explicit consent, or as authorised by Union or Member State law. Where ADM is permitted, Article 22(3) requires that data subjects have the rights to obtain ex post human intervention, to express their point of view, and to contest the decision.
The interpretation of key terms within Article 22(1) has led to debate about what constitutes a ‘decision’, the criteria for the decision to be recognised as solely automated, the scope of ‘legal effects’ and ‘similarly significant effects’, and whether non-personal data processing falls within the ambit of Article 22 (Bygrave, 2020; Binns and Veale, 2021; Mendoza and Bygrave, 2017). To address these ambiguities, the European Data Protection Board (EDPB) and its predecessor, the WP29, issued Guidelines on Automated Individual Decision-Making and Profiling (‘the Guidelines’), containing explanations and practical examples to ensure uniform interpretation of GDPR provisions related to ADM (WP251 Guidelines, 2017).
The Guidelines highlight the need for any ADM to be infused with meaningful human intervention to avoid being classified as fully automated, and further caution against attempts to circumvent the regulation through superficial human involvement. To qualify as meaningful, human involvement must be accompanied by the authority and competence to alter the decision. Simply applying automated profiles to individuals without any substantial influence on the outcome, even if the process involves a human, still falls under the category of ADM. The Guidelines stress that human decision-makers must consider multiple sources of information to mitigate the risks associated with overreliance on automated outcomes. These instructions are equally relevant to human interventions performed ex ante and ex post (Article 22(1) and 22(3)).
It must be noted, however, that the deployment of human agents does not automatically ensure consideration of data subjects’ interests or strengthen protection of their fundamental rights; data controllers may fail to establish adequate requirements for and controls of human decision-making (HDM), and the personal characteristics of human decision-makers may not be appropriate to promote these ends. Besides possibly having low qualifications and a lack of knowledge and experience, human agents may demonstrate a wide range of deficient behaviours when interacting with ADM, based on their own preconceptions[MOU2] , such as undue aversion to or excessive trust in ADMSs, and may also introduce their own bias, potentially compromising the decisions they are meant to oversee (Kern et al., 2022, Logg et al., 2019, Burton et al., 2019, Alexander et al., 2018).
Data controllers and the quality of human intervention in ADM
The core tenet of the GDPR’s approach is data controller accountability (GDPR: Article 5(2)). Enshrined within Articles 5 and 24, this responsibility mandates data controllers to demonstrate compliance through robust technical and organisational measures. Article 25(1) further mandates integrating data protection measures from the outset of processing activities, and Article 35 necessitates data protection impact assessments (DPIAs) where ADM is present, enabling a systematic evaluation of potential risks and countermeasures (Kaminski and Malgieri, 2021: 140). Additionally, Article 37 requires the appointment of data protection officers (DPOs) under certain circumstances to oversee and monitor data controllers’ compliance, and the DPO may be instrumental in ensuring meaningfulness of HDM (Sartor and Lagioia, 2020).
Within the GDPR framework, data controllers are entrusted with specific responsibilities concerning ADM. As stipulated in Article 22 of the GDPR, they bear responsibility for either implementing a meaningful human review process for automated outcomes, intended to shelter data subjects from exposure to ADM (to comply with the Article 22(1) prohibition), or offering a human review of an adopted automated decision (as permitted by Article 22(2)) on the data subject’s request (to comply with Article 22(3)). The procedures and processes introduced by data controllers to ensure the required human intervention may vary, as long as they guarantee the intervention’s meaningfulness (Almada, 2021; Wagner, 2019).
Lazcoz and De Hert argue that while being a compliance requirement, human intervention is also instrumental in ensuring accountability: data controllers are ultimately responsible for the automated decisions (Lazcoz and De Hert, 2022). But while the GDPR makes data controllers accountable for the implementation of the appropriate measures, including meaningful human intervention, it does not impose quality requirements for HDM which forms part of the overall HADM. The primary aim of the GDPR is to protect data subjects, and we argue the inclusion of duly considered, documented, and qualified HDM alongside ADM in the accountability framework is essential to strengthen data-subject protection and good governance.
ADM’s evolution prompts us to strike a delicate balance between the innovative potential of technology and the safeguarding of fundamental rights, and human decision-makers emerge as pivotal agents in this equilibrium. However, human involvement immunises neither ADM, nor HADM from challenges such as bias, discrimination, or lack of explainability or justification (Kern, 2022; Yeung, 2017). Given the necessity for human agents to engage meaningfully in the decision-making process or to meaningfully intervene upon the request of the data subject and considering their role in facilitating the accountability of data controllers, it is prudent for data controllers to integrate quality requirements for HDM into various aspects of the ADM-related accountability framework.
Firstly, recognising that data controllers and their human decision-makers are not equivalent, data controllers could enforce, as part of their organisational measures, internal policies and procedures addressing human decision-makers who act as agents for data controllers. In addition to the measures that ensure the presence of a human reviewer over ADM, these would cover reviewers’ qualifications and competencies, require specific skills and performance standards, and include assessment criteria and procedures relating to human decision-makers. Including human decision-makers in this manner is compulsory whether or not decision-making processes amount to ADM: either to justify the absence of ADM or to establish the mechanisms and substance of HDM upon request. Additionally, since the GDPR’s overarching principles and requirements apply to partly automated data processing (in contrast to fully automated decisions covered by Article 22), such inclusion of human agents enhances overall compliance and accountability of data controllers through the privacy by design framework.
Furthermore, the GDPR introduces the obligation for data controllers to conduct DPIAs when ADM is involved (WP251 Guidelines, 2017). These assessments extend beyond risk evaluation: they encapsulate a comprehensive overview of measures intended to mitigate risks and demonstrate GDPR compliance, considering the rights and interests of data subjects and other persons concerned. While a DPIA is likely to describe risks to data subjects and safeguarding measures in place, including envisaged human intervention, we argue that, as in the case of organisational measures, it must also include an assessment (pertinent requirements and performance review) of human intervenors themselves.
Lastly, Article 37 of the GDPR introduces one more human role on the data controller’s side – a data protection officer (DPO). As independent overseers of data controllers’ compliance with advisory powers, DPOs could contribute to the protection of data subjects by reinforcing the accountability framework and adding an independent perspective to ADM governance, offering guidance to the data controller on DPIAs and subsequently monitoring implementation, and dealing with data subjects’ inquiries related to the processing of their personal data and their rights.
While the existence of ADM does not necessarily require the appointment of a DPO, DPOs may serve as a twofold control point within the regulatory framework of the GDPR and ADM processes. Their knowledge of the data controller’s operations and processing practices related to ADM equips them to facilitate effective communication and cooperation with regulatory bodies. In essence, DPOs could bridge the gap between internal governance and external regulatory control (European Data Protection Board, 2024), enhancing transparency and accountability in the realm of ADM. On the other hand, as internal monitors for data controllers, DPOs are positioned to ensure that controllers incorporate quality requirements and scrutiny procedures into their operational processes for human intervention in ADM, thereby promoting better governance and ensuring that human intervenors interact with ADMSs in ways that benefit data subjects (Roig, 2017). Even at this level of expertise, however, it must be noted that the advantages of a DPO are conditional on their personal competence and their understanding of the changing technologies they work with (ibid.).
Data subjects and human decision-making
Under the GDPR, data subjects are entitled to human review by default (where ADM is prohibited per Article 22(1)) and, where ADM is permissible, upon their request (as per Article 22(3)). This human review must be meaningful, involving the consideration of other sources of information and carried out by an authorised human reviewer (WP251 Guidelines, 2017: 21). Additionally, when ADM is permitted, data subjects possess the right to voice their opinions and challenge automated decisions (Article 22(3)). Although Article 22(3) doesn’t explicitly clarify whether expressing viewpoints or contesting decisions should be directed toward an automated or human reviewer, the ultimate arbiter on ADM could not logically be a further layer of ADM. In other words, a human reviewer is required in all circumstances. Data subjects, however, have to rely on the HDM provided, with no influence over its quality.
Data subjects also have the right to be informed about ADM as articulated in Articles 13(2)(f) and 14(2)(g). This right entails being told about the existence of ADM in the data controller’s processing practices and receiving meaningful insight into the logic underpinning ADM processes, as well as their significance and potential implications for the data subject. Although the Guidelines recommend that data subjects should be informed even if automated decisions don’t fall under Article 22(1), data controllers might opt not to provide information to data subjects, exploiting a narrow interpretation of GDPR requirements which would see ADM accompanied by HDM fall outside the scope of Article 22(1) and hence outside the requirement to inform data subjects of the existence of ADM (WP251 Guidelines, 2017: 25). Detecting ADM under such circumstances could prove challenging at best. Without information on ADM or the manner of its implementation, non-compliance will effectively be impossible to detect, preventing the data subject from exercising their rights and impeding enforcement actions (Sivan-Sevilla, 2024; Lynskey, 2023).
Even when data subjects are aware of the application of ADM to their personal data, determining what exactly constitutes the ‘meaningful’ information data controllers should give is problematic; the issue of ADM explainability has been extensively debated by researchers and EU institutions (Bauer et al., 2021; Cobbe et al., 2021; Malgieri, 2021; Selbst and Powles, 2017). Here, we merely note that for data controllers to provide meaningful information, they must possess such information in the first place, which is not necessarily the case. Data controllers and their human reviewers of ADMSs may lack the capability to comprehend or access information about the operations and decision-making processes of ADMSs. As a result, they might be unable to furnish data subjects with the required information (Grant et al., 2023).
There are thus multiple factors in play which may expose data subjects to violations of their fundamental rights: the responsibility for ensuring meaningful human intervention lies with data controllers; there is potential for decisions to be disqualified from Article 22(1), with a corresponding lack of ADM information provision; and there may be enforcement bottlenecks. There is also the possibility that data controllers might prioritise compliance with data protection regulations over genuine data and data subject safeguarding, leading to a risk of ‘compliance washing’ on their part, notably in the context of ADM and requisite human intervention.
Our contention is that given these potential difficulties, it would be more logical to address hybrid HADM, informing data subjects about the existence of ADM under all circumstances, and augmenting the current legal requirements by providing information about the extent of HDM and the fundamentals of ADM–HDM interaction involved in a given situation. Notifying data subjects about ADM is crucial for transparency, and this extra emphasis on HDM could enhance accuracy and trustworthiness by making it evident to data subjects that ADM and HDM are two facets of the same decision-making process.
This could potentially be facilitated through the existing pathways available under the GDPR, such as the right of access and data protection impact assessments (DPIAs). However, it’s crucial to recognise that these pathways come with inherent limitations that must be acknowledged and addressed to ensure effective transparency and accountability.
While data controllers must provide the information concerning ADM at or around the point when personal data is collected (Articles 13 and 14), data subjects retain the right to access (request) equivalent information at any time (Article 15). The information provided under Article 15 may need to be updated or customised to reflect processing operations specific to the requesting data subject (Custers and Heijne, 2022). This distinction between the duty to provide information and the right to access it is intended to enable data subjects to verify the accuracy of the data and the lawfulness of the processing.
Data subjects who exercise their right of access can gain extra insights into ADM, including information about inferred or derived data, such as algorithmic outcomes or personalised results, and details about the rationale behind decisions made about them personally, rather than about ADM logic in general (Custers and Heijne, 2022: 5). The question remains, however, as to whether giving a particular data subject information about a specific decision actually contributes to assessing the fairness, impartiality, and legitimacy of that decision. Given that the data subject is unlikely to be privy to analogous decisions concerning other individuals for validation of decision-making consistency or identification of factors driving decision disparities, this issue remains arguable (Dreyer and Schulz, 2019). On the face of it, the right of access bolsters individual data protection, while also fostering the pursuit of social equity and public interest when exercised collectively in the dimension of civil society, though there has been little uptake of this possibility so far (Mahieu and Ausloos, 2020a).
DPIAs mandated by the GDPR offers an additional avenue for data subjects to engage in ADM governance: data controllers can ask for the opinions of data subjects (and their representatives) on intended processing. However, data subjects might be sidelined if data controllers perceive seeking their input as inappropriate, particularly if commercial or public interests or the security of processing operations are at stake (GDPR: Article 35(9)). Another challenge associated with this mechanism is that consultations might devolve into mere formalities due to factors such as DPIA design and content choices, individuals’ capacities, and their willingness to develop a detailed grasp of the subject matter or actively participate in the DPIA (Christofi et al., 2022). In light of this, designating a data-subject representative – such as a nonprofit organisation or an association specialising in data subject protection – could serve as a more effective solution, advocating for data subjects’ interests in the manner of a consumer protection group. Although various advocacy entities are already operating, their capacity and resources remain limited (Mahieu and Ausloos, 2020b).
Again, in situations where DPIAs encounter challenges in effectively engaging data subjects or ensuring their interests are adequately represented, a DPO could be instrumental. By ensuring compliance with GDPR requirements and advocating for the rights of data subjects, the DPO can help address potential shortcomings in the DPIA process and enhance its effectiveness in safeguarding data subjects’ interests.
The AI Act: providers, deployers, and affected persons
AI Act human oversight and GDPR human intervention
The AI Act (AIA) introduces additional regulatory measures pertaining to ADM and intersects with the GDPR on several fronts. While the GDPR initially aspired to be a technology-agnostic framework, the AIA explicitly targets AISs, and its overarching objective is to establish a legal framework in which AI systematically prioritises humans (AI Act: Recital 6). The AIA designates human agency (serving people) and human oversight (appropriate supervision by humans) as the primary guiding principles for the development and use of AI at all levels of risk (AI Act: Recital 27).
The AIA also covers human oversight requirements and obligations in relation to high-risk AISs – those which pose significant risk of harm to the health, safety, or fundamental rights of natural persons, and which might therefore also fall under the scope of Article 22 of the GDPR – to the extent such systems produce decisions with legal or similarly significant effects on data subjects. As the AIA will apply concurrently with the GDPR, these requirements for high-risk AISs together with the obligations of providers and deployers will contribute to the protection of citizens in the ADM realm, as well as to data-controller accountability under the GDPR umbrella (AI Act: Article 2(7)).
The notion of human oversight within the AIA appears to encompass a broader scope yet be less precisely defined than the idea of human intervention outlined in the GDPR (Lazcoz and De Hert, 2022: 12). While the GDPR aims to separate decision targets from ADM by using meaningful human lenses, the AIA requires that the design of AISs incorporate human-machine interaction tools which allow a human to oversee the systems adequately in relation to the associated risks (AI Act: Article 14). Human intervention under Article 22 of the GDPR might be an example of such human oversight.
Article 14 of the AIA mandates that high-risk AISs be equipped with appropriate human-machine interface tools, ensuring oversight by natural persons. The primary objective of human oversight is the mitigation of risks inherent to AISs, including those affecting fundamental human rights, and the oversight must consider the specific risks, the level of autonomy, and the context of the AIS (AI Act: Article 14(2) and 14(3)). Providers bear responsibility for enabling individuals assigned to undertake human oversight to understand the capacities and limitations of the high-risk AIS they are overseeing, interpret its outcomes, intervene in its operation, decide on whether (or not) to use it and remain aware of the tendency to blindly rely or over rely on its results (automation bias) (AI Act: Article 14(4)).
Article 14(4) prompts inquiry into the feasibility of ensuring that human overseers maintain awareness, comprehension, and accurate interpretation of system operations and outputs. This entails a consideration of various factors such as the personal characteristics, working conditions, and cognitive abilities of those tasked with oversight (Koivisto et al., 2024). While AISs providers may fulfil their obligation by providing the requisite information and implementing designated oversight measures, these measures do not in themselves answer the question of whether human overseers can effectively fulfil their roles.
AI system deployers: human oversight and human overseers
Human actors are engaged throughout the entire operational cycle of an AIS, from the initial decision to deploy the system, through its use phase, to the application of its outputs in specific cases. The degree of human involvement varies, with common approaches such as ‘human-in-the-loop’ (active human engagement) and ‘human-on-the-loop’ (supervision by humans) being widely implemented. Regardless of the level of autonomy an AIS might possess, the presence of humans, albeit to varying degrees, remains an integral aspect of its operation.
Recent research advocates for viewing human-algorithm interactions as a form of collaborative agency rather than treating human and machine functions in isolation (Tsamados et al., 2024; Green, 2022). While serving as a front-line control mechanism, prescribed human oversight constitutes an integral component of the HADM nexus. However, the effectiveness of this oversight depends on the skills and motivation of the overseers.
Article 26 of the AIA requires deployers to implement human oversight measures in alignment with the instructions supplied by the provider1 and ensure that individuals assigned to carry out human oversight have the competence, authority, support, and training needed to oversee AISs effectively. Additionally, deployers must undertake proactive measures to cultivate a sufficient level of AI literacy among their personnel involved in the operation and utilisation of AISs (AI Act: Article 4). These measures must be tailored to individual technical knowledge, experience, education, training, and the specific contexts in which AISs are deployed.
The AIA’s stipulations regarding AI literacy and necessary training assume profound significance, though they remain somewhat limited in scope. As deployers are not explicitly required to incorporate their human overseers into the accountability framework to ensure that these individuals genuinely possess the requisite competence, the requirements relating to their quality risk existing only on paper.
Meaningful and qualified human oversight, acknowledged as a vital component in safeguarding the interests of individuals and society, should be integrated into the governance model of deployers when employing HADM. This integration would require establishing clear quality standards for human overseers, encompassing both technical expertise and personal attributes relevant to their role within the deployer’s operational context (Tsamados et al., 2024; Laux, 2023). It would also require ongoing evaluation of the performance of human decision-makers in practice, ensuring that they apply their knowledge and skills effectively, avoid the twin dangers of overreliance or reluctance in interacting with ADMS, and do not compromise the overall performance of HADM.
By establishing criteria for competence, knowledge, and moral character, and by integrating these criteria with existing legal requirements, the governance models of deployers would align with the proclaimed comprehensive and context-aware approach to AI governance, ultimately contributing to the protection of affected individuals and society.
AI system deployers: fundamental rights impact assessment and HADM governance
Earlier, we discussed DPIAs as a promising tool for data controllers tasked with governing HADM. We will now explore how HADM governance model can be enhanced under the AIA’s framework, suggesting ways to incorporate human overseers into such model.
The AIA mandates deployers of certain high-risk AISs to produce fundamental rights impact assessments (FRIAs), detailing the AIS’ operational context, potential risks, and also the safeguarding measures, including human oversight, which the deployer will put in place as an element of the risk mitigation framework (AI Act: Article 27). In the ADM context, DPIAs and FRIAs must be produced in conjunction, and may come under public scrutiny where deployers are obliged to publish their FRIAs (AI Act: Article 27(4)). Together, these assessments could serve as a robust safeguarding tool ensuring the transparency and quality of HADM (Mantelero, 2022).
Since the AIA imposes enhanced requirements on individuals assigned with human oversight, including their AI literacy (competence, knowledge, and skills), FRIAs could serve as a framework for more comprehensive and all-encompassing evaluation which considers human agents’ abilities and competencies, and their capacity to function effectively alongside AISs as an integral part of HADM. Human overseers must supervise not only the operation of AISs but also mitigate risks associated with human intervention itself, such as biases, errors, and overreliance on AISs. However, the AIA’s provisions primarily focus on the assessment of AISs’ risks and overlook the intricate dynamics of HADM, neglecting to ensure the quality of HDM. Deployers need clearer guidelines for assessing the competence and readiness of their human overseers.
This can be achieved through various pathways. Deployers must demonstrate accountability by developing internal policies that could explicitly outline the qualifications, competencies, and responsibilities of human overseers, and how they will monitor ADMSs, intervene and report their findings, and contribute to HADM (Crootof et al., 2023). FRIAs could aid in establishing an adequate HADM governance framework that clearly defines relevant roles, responsibilities, and requisite standards for both HDM and ADM, enhancing transparency and accountability.
FRIAs could require detailed job descriptions and periodic assessments of human overseers. These assessments would ensure that human decision-makers are not only well-versed in the technical and operational aspects of AISs but also capable of critically assessing the implications of HADM dynamics and outputs (Sterz et al., 2024; Enarsson et al., 2021). FRIAs could frame ongoing training for overseers to maintain pertinent technical expertise and awareness of legal and ethical standards related to ADM.
HADM governance should include mechanisms for tracing and auditing HDM inputs. Human overseers, enabled to intervene in or override automated decisions, should be capable of explaining the rationale and anticipated consequences of their actions. Moreover, human overseers should be able to recognise and mitigate their own biases and operate with integrity, prudence, impartiality, sound moral judgement, and benevolence.
FRIAs could continuously assess and validate HADM governance measures, as well as how human decision-makers interact with ADMSs, auditing the quality of HDM performance. Furthermore, FRIAs could incorporate provisions for seeking second opinions, including from the DPO, to add an additional layer of scrutiny. By mandating such measures, FRIAs could ensure that human overseers are held to the same rigorous standards as the ADMSs they oversee.
Additionally, deployers could establish feedback mechanisms to allow overseers to report issues or concerns, identifying potential areas for improvement in the ADMS or governance framework. Establishing whistle-blower protections and anti-retaliation safeguards could support workers who challenge ADM or report system failures, ensuring they are not deterred by fears of job insecurity or management repercussions.
Affected persons and their rights
Although the AIA does not seem to improve the potential for collective redress mechanisms, which was a limitation of the GDPR discussed earlier, it does include additional safeguards for individuals. According to Article 26(11) of the AIA, individuals subject to the use of high-risk AISs which make or assist in decisions in relation to them must be informed that they are subject to use of such AISs. Although the AIA only requires notification of the use of such AISs, and not the provision of additional information about its implications for individuals as required by Articles 13 and 14 of the GDPR, its wording extends beyond the scope of the right to be informed under the GDPR, explicitly requiring communication of the use of decision-assisting AISs (i.e. even if HDM exists). It also broadens the range of individuals granted the right to be informed, in that it does not distinguish between personal and non-personal data and is not specifically restricted to data subjects.
This, nonetheless, may be undermined by the exclusion of AISs from the high-risk category if they do not have a ‘material’ impact on decisional outcomes and do not pose significant risks to the health, safety, or fundamental rights of individuals (as outlined in Article 6(3) of the AIA). The ‘materiality’ qualifier appears subjective, potentially allowing for circumvention of the requirements laid down for high-risk AISs.
The AIA further stipulates that individuals subject to the use of high-risk AISs must have ‘the right to obtain from the deployer clear and meaningful explanations of the role of the AIS in the decision-making procedure and the main elements of the decision taken’. This provision holds out the prospect that, if provided with such information, individuals could potentially reverse-engineer decisions, thereby uncovering the role of HDM. However, this process would require a willingness to undertake such analysis, something which might be of greater interest to auditors, data protection organisations, or supervisory authorities than to individuals.
The AIA attempts to ensure that the humans overseeing high-risk AISs on behalf of deployers can correctly interpret their outputs. The intent of this interpretability requirement is to guarantee that deployers are capable of providing the mandated explanation of the automated decision, but how ‘correct’ interpretation is guaranteed and what ‘clear and meaningful explanation’ involves remain to be seen.
Conclusion
Human control is widely regarded as essential for mitigating risks associated with automated decision-making systems (ADMSs). This article has critically engaged with the human oversight concept, challenging the assumption that human control is inherently good without a rigorous examination of its quality. We advocate for a holistic perspective on human-automated decision-making (HADM), treating algorithmic and human agents as integral components of HADM to ensure that ADMSs best serve individuals and civil society.
Our findings highlight that while the GDPR and the AIA offer foundational frameworks for embedding human oversight within ADMSs, they fall short in addressing the qualitative dimensions of this oversight. The GDPR mandates human intervention in certain ADM processes but lacks specific requirements for the qualifications or training of human overseers. Similarly, the AIA acknowledges the need for AI literacy for those interacting with ADMSs but does not detail guidelines for quality standards and ongoing evaluation of their performance.
To bridge these gaps, a comprehensive governance strategy is necessary. This should include regular performance reviews, continuous feedback mechanisms, and ongoing professional development for human overseers. Assessments should address not only the accuracy and fairness of decisions but also the effectiveness of risk management and adherence to ethical standards. Feedback mechanisms, including anonymous channels, are essential for building a culture of transparency and continuous improvement. Moreover, continuous training would help overseers remain informed and adept at operating ADMSs.
Regulatory instruments such as data protection and fundamental rights impact assessments must be leveraged more effectively to include requirements for and assessments of both ADM and HDM. This dual focus ensures a holistic approach to risk management, where the human element of HADM is equally subject to continuous monitoring and evaluation to mitigate potential biases and maintain decision-making quality. This approach aligns with the concept of adaptive governance, which posits that oversight mechanisms must evolve in response to changing conditions and new information.
However, we must acknowledge several limitations. Implementing such a strategy may face practical challenges, including organisational, economic, and human resource constraints. The effectiveness of feedback mechanisms relies on the willingness of human overseers to engage openly, which can be influenced by organisational culture and power dynamics. Furthermore, without legal mandates for specific measures, HADM deployers might only meet minimum compliance standards, neglecting complementary safeguards.
In conclusion, addressing the challenges of human oversight in ADMSs requires a multifaceted approach involving quality standards, ongoing training, continuous feedback, and performance assessments. Despite the lack of clear authoritative guidance on the governance of the human element within HADM, deployers should incorporate specific qualifications for human agents, their interactions with ADMSs, and mechanisms for accountability and transparency into their governance models. Human agents cannot be an afterthought or a mere checkbox in compliance; they must be seen and actively managed as an integral component of HADM processes. Only through such comprehensive governance can a robust and trustworthy HADM environment be ensured.
Bibliographical references
AEPD. “Evaluating human intervention in automated decisions”. 4 March 2024. Available at: https://www.aepd.es/en/press-and-communications/blog/evaluating-human-intervention-in-automated-decisions [online] [Date accessed: 01.09.2024]
Alexander, Veronika, Blinder, Collin, and Zak, Paul J. “Why trust an algorithm? Performance, cognition, and neurophysiology”. Computers in Human Behavior, 89 (2018), p. 279–288. DOI: https://doi.org/10.1016/j.chb.2018.07.026 [Date accessed: 01.09.2024]
Almada, Marco. “Human Intervention in Automated Decision-Making”, in Proceedings of the Seventeenth International Conference on Artificial Intelligence and Law. New York: ACM (2019) p. 2–11. DOI: https://doi.org/10.1145/3322640.3326699 [Date accessed: 01.09.2024]
Almada, Marco. “Automated Decision-Making as a Data Protection Issue”. Social Science Research Network, 2021 [online]. DOI: https://doi.org/10.13140/RG.2.2.14603.21289 [Date accessed: 01.09.2024]
Bauer, Kevin, Hinz, Oliver, van der Aalst, Wil and Weinhardt, Christof. “Expl(AI)n It to Me – Explainable AI and Information Systems Research”. Business and Information Systems Engineering, 63 (2021), p. 79–82. DOI: https://doi.org/10.1007/s12599-021-00683-2 [Date accessed: 01.09.2024]
Berger, Armin, Hillebrand, Lars, Leonhard, David et al. "Towards Automated Regulatory Compliance Verification in Financial Auditing with Large Language Models". 2023 IEEE International Conference on Big Data (BigData), Sorrento, Italy (2023), p. 4626–4635. DOI: https://doi.org/10.1109/BigData59044.2023.10386518 [Date accessed: 01.09.2024]
Berger, Benedikt, Adam, Martin, Rühr, Alexander and Benlian, Alexander. “Watch Me Improve: Algorithm Aversion and Demonstrating the Ability to Learn”. Business and Information Systems Engineering, 63 (2021), p. 55–68. DOI: https://doi.org/10.1007/s12599-020-00678-5 [Date accessed: 01.09.2024]
Binns, Reuben and Veale, Michael. “Is that your final decision? Multi-stage profiling, selective effects, and Article 22 of the GDPR”. International Data Privacy Law, 11(4) (2021), p. 319–332. DOI: https://doi.org/10.1093/idpl/ipab020 [Date accessed: 01.09.2024]
Burton, Jason W., Stein, Mari-Klara, and Jensen, Tina Blegind. “A systematic review of algorithm aversion in augmented decision making”. Journal of Behavioral Decision Making, 33(2) (2019), p. 220–239. DOI: https://doi.org/10.1002/bdm.2155 [Date accessed: 01.09.2024]
Bygrave, Lee A. “Article 22: Automated individual decision-making, including profiling”, in Kuner, Christopher, Bygrave, Lee A., Docksey, Christopher and Dreachsler, Laura (eds), The EU General Data Protection Regulation (GDPR) – A Commentary. Oxford: Oxford University Press, p. 531. (2020) DOI: http://dx.doi.org/10.2139/ssrn.3839645 [Date accessed: 01.09.2024]
Christofi, Athena, Breuer, Jonas, Wauters, Ellen et al. “Data protection, control and participation beyond consent - Seeking the views of data subjects in data protection impact assessments”, in Kosta, E. and Leenes, R. (eds), Research Handbook on EU Data Protection Law, Cheltenham: Edward Elgar, 2022, p. 503–529. (2022) DOI: https://doi.org/10.4337/9781800371682.00029 [Date accessed: 01.09.2024]
Cobbe, Jennifer, Seng Ah Lee, Michelle and Singh, Jatinder. “Reviewable Automated Decision-Making: A Framework for Accountable Algorithmic Systems”, in Proceedings of the 2021 ACM Conference on Fairness, Accountability, and Transparency (FAccT ‘21). New York: ACM (2021), p. 598–609. DOI: https://doi.org/10.1145/3442188.3445921 [Date accessed: 01.09.2024]
Crootof, Rebecca and Kaminski, Margot E. and Price II, William Nicholson. Humans in the Loop (March 25, 2022). 76 Vanderbilt Law Review 429 (2023), U of Colorado Law Legal Studies Research Paper No. 22-10, U of Michigan Public Law Research Paper No. 22-011, DOI: http://dx.doi.org/10.2139/ssrn.4066781 [Date accessed: 01.09.2024].
Custers, Bart and Heijne, Anne-Sophie. The Right of Access in Automated Decision-Making: The Scope of Article 15(1)(h) GDPR in theory and practice. Computer Law and Security Review, (2022) DOI: https://doi.org/10.1016/j.clsr.2022.105727
Dreyer, Stephan and Schulz, Wolfgang. “The General Data Protection Regulation and. Automated Decision-making: Will it deliver?”. Discussion Paper Ethics of Algorithms #5, Bertelsmann Stiftung (2019). DOI: https://doi.org/10.11586/2018018 [Date accessed: 01.09.2024]
Edwards, Lilian and Veale, Michael. " Enslaving the Algorithm: From a ‘Right to an Explanation’ to a ‘Right to Better Decisions’? IEEE Security & Privacy, 16(3) (2018), 46–54. DOI: https://doi.org/10.1109/MSP.2018.2701152 [Date accessed: 01.09.2024].
Enarsson, Therese, Enqvist, Lena and Naarttijärvi, Markus. Approaching the human in the loop – legal perspectives on hybrid human/algorithmic decision-making in three contexts. Information & Communications Technology Law, 31(1) (2021), p. 123–153. DOI: https://doi.org/10.1080/13600834.2021.1958860 [Date accessed: 01.09.2024].
Enqvist, Lena. “‘Human oversight’ in the EU artificial intelligence act: what, when and by whom?”. Law, Innovation and Technology, 15(2) (2023), p. 508–535. DOI: https://doi.org/10.1080/17579961.2023.2245683 [Date accessed: 01.09.2024]
European Data Protection Board, Coordinated Enforcement Action, Designation and Position of Data Protection Officers (adopted January 2024). Available at: https://www.edpb.europa.eu/our-work-tools/our-documents/other/coordinated-enforcement-action-designation-and-position-data_en [Date accessed: 01.09.2024]
European Parliament. “Regulation (EU) 2024/1689 of the European Parliament and of the Council of 13 June 2024 laying down harmonised rules on artificial intelligence and amending Regulations (EC) No 300/2008, (EU) No 167/2013, (EU) No 168/2013, (EU) 2018/858, (EU) 2018/1139 and (EU) 2019/2144 and Directives 2014/90/EU, (EU) 2016/797 and (EU) 2020/1828 (Artificial Intelligence Act) (Text with EEA relevance)”. Official Journal of the European Union, L series, 2024/1689, (12 July 2024) (online) http://data.europa.eu/eli/reg/2024/1689/oj
Grant, David Gray, Behrends, Jeff and Basl, John. “What we owe to decision-subjects: beyond transparency and explanation in automated decision-making”. Philosophical Studies, (2023). DOI: https://doi.org/10.1007/s11098-023-02013-6 [Date accessed: 01.09.2024]
Green, Ben. “The flaws of policies requiring human oversight of government algorithms”. Computer Law & Security Review, Volume 45 (2022), DOI: https://doi.org/10.1016/j.clsr.2022.105681 [Date accessed: 01.09.2024]
Kaminski, Margot E. and Malgieri, Gianclaudio. “Algorithmic impact assessments under the GDPR: Producing multi-layered explanations”. International Data Privacy Law, 11(2) (2021), p. 125–144. DOI: https://doi.org/10.1093/idpl/ipaa020 [Date accessed: 01.09.2024]
Kern, Christoph, Gerdon, Frederic, Bach, Ruben L. et al. “Humans versus machines: Who is perceived to decide fairer? Experimental evidence on attitudes toward automated decision-making.” Patterns, 3(10) (2022). DOI: https://doi.org/10.1016/j.patter.2022.100591 [Date accessed: 01.09.2024]
Koivisto, Ida, Koulu, Riikka, and Larsson, Stefan. User accounts: How technological concepts permeate public law through the EU’s AI Act. Maastricht Journal of European and Comparative Law, 0(0) (2024). DOI: https://doi.org/10.1177/1023263X241248469 [Date accessed: 01.09.2024]
Koulu, Riikka. Proceduralizing control and discretion: Human oversight in artificial intelligence policy. Maastricht Journal of European and Comparative Law, 27(6) (2020), p. 720-735. DOI: https://doi.org/10.1177/1023263X20978649 [Date accessed: 01.09.2024]
Kyriakou, Kyriakos, Otterbacher, Jahna. In humans, we trust. Discover Artificial Intelligence, 44 (2023). DOI: https://doi.org/10.1007/s44163-023-00092-2 [Date accessed: 01.09.2024]
Lazcoz, Guillermo and De Hert, Paul. “Humans in the GDPR and AIA governance of automated and algorithmic systems. Essential pre-requisites against abdicating responsibilities”. VUB Brussels Privacy Hub Working Paper, vol. 8 no 32 (2022). DOI: https://doi.org/10.2139/ssrn.4016502 [Date accessed: 01.09.2024]
Logg, Jennifer M., Minson, Julia A., Moore Don A. “Algorithm appreciation: people prefer algorithmic to human judgment”. Organizational Behavior and Human Decision Processes, 151 (2019), p. 90–103. DOI: https://doi.org/10.1016/j.obhdp.2018.12.005 [Date accessed: 19.03.2024]
Lynskey, Orla. “Regulating for the Future: The Law’s Enforcement Deficit”. Studies: An Irish Quarterly Review, 112(445) (2023), p. 104–119. DOI: https://doi.org/10.1353/stu.2023.0007 [Date accessed: 01.09.2024]
Mahieu, René L. P. and Ausloos, Jef (2020a). “Recognising and Enabling the Collective Dimension of the GDPR and the Right of Access”, Law Archive, 2 July 2020. DOI: https://doi.org/10.31228/osf.io/b5dwm [Date accessed: 01.09.2024]
Mahieu, René L. P. and Ausloos, Jef (2020b). “Harnessing the collective potential of GDPR access rights: towards an ecology of transparency”. Internet Policy Review, 6 July 2020. Available at: https://policyreview.info/articles/news/harnessing-collective-potential-gdpr-access-rights-towards-ecology-transparency/1487 [Date accessed: 01.09.2024]
Malgieri, Gianclaudio. “‘Just’ Algorithms: Justification (Beyond Explanation) of Automated Decisions Under the General Data Protection Regulation”. Law and Business, 1(1) (2021), p. 16-28. DOI: https://doi.org/10.2478/law-2021-0003 [Date accessed: 01.09.2024]
Mantelero, Alexander. “Beyond Data”, in Beyond Data (Information Technology and Law Series 36). The Hague: T.M.C Asser Press, p. 1–43. (2022) DOI: https://doi.org/10.1007/978-94-6265-531-7_1 [Date accessed: 01.09.2024]
Mendoza, Isak and Bygrave, Lee A. “The Right not to be Subject to Automated Decisions based on Profiling”, in T. E. Synodinou, P. Jougleux, C. Markou and T. Prastitou (eds), EU Internet Law: Regulation and Enforcement. Cham: Springer, p. 77–98 DOI: https://doi.org/10.1007/978-3-319-64955-9_4 [Date accessed: 01.09.2024]
Misuraca, Gianluca and van Noordt, Colin. AI Watch: Artificial Intelligence in public services, EUR 30255 EN. Luxembourg: Publications Office of the European Union, Luxembourg, 2020. DOI: https://doi.org/10.2760/039619, JRC120399 [Date accessed: 01.09.2024]
Novelli, Claudio, Taddeo, Mariarosaria and Floridi, Luciano. Accountability in artificial intelligence: what it is and how it works. AI & Soc 39, 1871–1882 (2024). DOI: https://doi.org/10.1007/s00146-023-01635-y [Date accessed: 01.09.2024]
Roig, Antoni. “Safeguards for the right not to be subject to a decision based solely on automated processing (Article 22 GDPR)”, European Journal of Law and Technology, 8(3) (2017). Available at: https://ejlt.org/index.php/ejlt/issue/view/51 [Date accessed: 01.09.2024]
Roig, Antoni. Las garantías frente a las decisiones automatizadas. Del Reglamento General de Protección de Datos a la gobernanza algorítmica. Barcelona: J.M. Bosch, 2020. DOI: https://doi.org/10.2307/j.ctv1dv0v54 [Date accessed: 01.09.2024]
Rovatsos, Michael, Mittelstadt, Brent and Koene, Ansgar. Landscape Summary: Bias in Algorithmic Decision-Making: What is bias in algorithmic decision-making, how can we identify it, and how can we mitigate it? UK Government (2019). Available at: https://www.gov.uk/government/publications/landscape-summaries-commissioned-by-the-centre-for-data-ethics-and-innovation [Date accessed: 01.09.2024]
Sartor, Giovanni and Lagioia, Francesca. The impact of the General Data Protection Regulation (GDPR) on artificial intelligence. Study for the European Parliament Panel for the Future of Science and Technology (STOA) (2020) Available at: https://www.europarl.europa.eu/thinktank/en/document/EPRS_STU(2020)641530 [Date accessed: 01.09.2024]
Schmidt, Philipp, Biessmann, Felix, and Teubner, Timm. “Transparency and trust in artificial intelligence systems”. Journal of Decision Systems, 29(4) (2020), p. 260–278. DOI: https://doi.org/10.1080/12460125.2020.1819094 [Date accessed: 01.09.2024]
Selbst, Andrew D. and Powles, Julia. “Meaningful information and the right to explanation”. International Data Privacy Law, 7(4) (2017), p. 233–242. DOI: https://doi.org/10.1093/idpl/ipx022 [Date accessed: 01.09.2024]
Sivan-Sevilla, Ido. “Varieties of enforcement strategies post-GDPR: a fuzzy-set qualitative comparative analysis (fsQCA) across data protection authorities”. Journal of European Public Policy, 31(2), (2024) p. 552–585. DOI: https://doi.org/10.1080/13501763.2022.2147578 [Date accessed: 01.09.2024]
Sterz, Sarah, Baum, Kevin, Biewer, Sebastian, Hermanns, Holger, Lauber-Rönsberg, Anne, Meinel, Philip, and Langer, Markus. On the quest for effectiveness in human oversight: Interdisciplinary perspectives. In Proceedings of the 2024 ACM Conference on Fairness, Accountability, and Transparency, (2024) pp. 2495–2507. Association for Computing Machinery. DOI: https://doi.org/10.1145/3630106.3659051 [Date accessed: 01.09.2024]
Tsamados, Andreas; Floridi, Luciano y Taddeo, Mariarosaria. “Human control of AI systems: from supervision to teaming”. AI Ethics, (2024). DOI: https://doi.org/10.1007/s43681-024-00489-4
Tsamados, Andreas; Floridi, Luciano and Taddeo, Mariarosaria. Human control of AI systems: from supervision to teaming. AI Ethics, (2024). DOI: https://doi.org/10.1007/s43681-024-00489-4
Wagner, Ben. “Liable, but Not in Control? Ensuring Meaningful Human Agency in Automated Decision‐Making Systems”. Policy and Internet, 11(1) (2019), p. 104–122. DOI: https://doi.org/10.1002/poi3.198 [Date accessed: 01.09.2024]
Wieringa Maranke. “Hey SyRI, tell me about algorithmic accountability”: Lessons from a landmark case. Data & Policy, 5:e2 (2023). DOI: https://doi.org/10.1017/dap.2022.39 [Date accessed: 01.09.2024]
WP251, European Data Protection Board. “Guidelines on Automated individual decision-making and Profiling for the purposes of Regulation 2016/679”, 17/EN WP251rev.01, (3 October 2017) (online) [Date accessed: 01.09.2024] https://ec.europa.eu/newsroom/article29/items/612053/en
Yeung, Karen. “Algorithmic Regulation: A Critical Interrogation”. Regulation and Governance, 12(4) (2018), p. 505–523. DOI: https://doi.org/10.1111/rego.12158 [Date accessed: 01.09.2024]
Notes:
1- As highlighted by Enqvist, this allocation of human oversight responsibilities might be weakened by the fact that deployers are required to follow the provider’s instructions, i.e. they might not develop human oversight beyond the scope of such instructions, while the measures identified by a provider might be insufficient or inadequate (Enqvist, 2023).
Key words: human oversight, General Data Protection Regulation (GDPR), artificial intelligence (AI), automated decision-making (ADM), accountability, European Union (EU)
How to cite this article: Levitina, Anna. «Los seres humanos en la toma de decisiones automatizada en el marco del RGPD y la Ley de IA». Revista CIDOB d’Afers Internacionals, n.º 138 (diciembre de 2024), p. 121-144. DOI: doi.org/10.24241/rcai.2024.138.3.121/en
Revista CIDOB d’Afers Internacionals, nº 138, pp. 121-144
Quadrimestral (September-December 2024)
ISSN:1133-6595 | E-ISSN:2013-035X
DOI: https://doi.org/10.24241/rcai.2024.138.3.121/en
Receipt date: 20.03.24 ; Acceptance date: 05.08.2024