Mapping urban artificial intelligence: first report of GOUAI’s Atlas of Urban AI
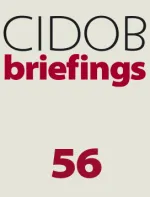
The Atlas of Urban AI, conceived as the flagship project of the Global Observatory of Urban Artificial Intelligence (GOUAI), has established itself as the largest and most comprehensive openly accessible repository of ethical urban artificial intelligence initiatives worldwide. Building on the research conducted, this report analyses the cases included in the Atlas and provides a comprehensive view of best practices and trends characterising the global evolution of urban AI.
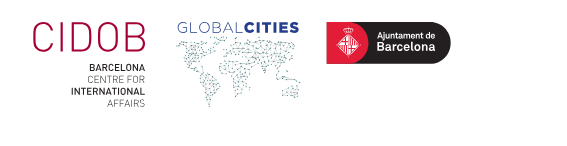
1. Introduction
Artificial intelligence (AI) stands as one of the most transformative forces of our era, carrying the promise to reshape our societies. Unsurprisingly, this phenomenon has captured the attention of governments worldwide, and local governments are no exception. A recent survey reveals a strong interest among mayors in exploring the use of AI tools to enhance public service delivery and address critical urban challenges such as traffic congestion, infrastructure upgrades, public safety and climate change mitigation. This keen interest is not merely theoretical: nearly 70% of the surveyed mayors reported testing this technology, albeit with only a minority actively deploying it. Therefore, the tangible impact of AI on urban areas is indisputably significant.
However, the enthusiasm surrounding the adoption of “urban AI” is tempered by an equal level of caution in embracing algorithmic tools. This caution stems from the potential impact of AI systems on rights, as well as the significant social risks and environmental costs associated with the increasing reliance of our societies and governments on AI systems. Furthermore, urban AI applications operate within specific policy contexts, thus wielding distinct political influence. Cities are political arenas where local governments have the authority to make decisions that impact millions of lives. It is no coincidence that the EU AI Act categorises certain common uses of AI systems in urban environments as either high-risk or limited-risk, making it imperative to scrutinise the interconnections between algorithmic systems and their ethical and political implications.
In essence, there is an urgent requirement for cities to acquire knowledge on the ethical deployment of AI. And this is precisely the mission that the Atlas of Urban AI aims to accomplish. Conceived as the flagship project of the Global Observatory of Urban Artificial Intelligence (GOUAI), the Atlas offers a curated collection of over 200 initiatives from 70 cities around the globe, establishing itself as the most comprehensive openly accessible repository of urban artificial intelligence worldwide. Building on the research conducted, this report seeks to analyse the cases included in the Atlas to provide an overall view of best practices and trends characterising the global evolution of urban AI.
Specifically, the report addresses the following questions: In which regions do local governments exhibit greater engagement in deploying AI systems with an ethical focus? What ethical principles do cities most frequently apply, and why? How are these principles operationalised and which ones tend to be overlooked? At what stage of implementation are municipalities in their deployment of AI technology? Are most projects already operational or is the emphasis still primarily on discussing pilot projects? What characterises the urban AI ecosystem for local governments, and who are their main partners? Is urban AI exclusive to larger cities?
The report is structured into four sections. The first section provides methodological insights into the research process behind the curation of the Atlas, including information on the criteria used to select the cases featured in it. The second section is more theoretical and aims to clarify the concept of “urban artificial intelligence”. It also reflects on the relationship between urban settings and artificial intelligence technologies and highlights distinguishing features that differentiate urban AI from other types of algorithmic systems. The third section presents key trends in urban artificial intelligence from the analysis of the Atlas. Finally, the fourth section explores the local regulation of AI and identifies policy initiatives undertaken by cities globally to ensure the ethical deployment of algorithmic systems.
2. Methodological note
GOUAI’s Atlas of Urban AI was built on extensive desk research, involving the systematic gathering of data from various publicly accessible sources. These include official city reports, government websites, urban development agencies, technology company reports, smart city newspapers and other related online platforms. To a lesser extent, interviews and surveys with city officials and other relevant stakeholders were also conducted. The research, initiated in 2021, is still in progress and remains open to receiving additional cases via an online survey.
Projects included in the database were required to meet four specific criteria, namely: (1) an explicit alignment with GOUAI’s ethical principles;1 (2) direct or indirect involvement and/or support of the city government; (3) a clear focus on urban AI (i.e. with identifiable applications in an urban context); and (4) a documented record of planning, implementation or active development.
Although this report and the extensive research supporting the Atlas strive to be thorough, the authors recognise possible omissions in their efforts to capture every case in the Atlas. This is due to significant limitations encountered during the data collection process. The main challenge stemmed from the heavy reliance on public sources, given that many cities do not actively promote their urban AI projects or provide easily accessible project information. This lack of promotion and transparency constitutes a key barrier to obtaining comprehensive and current data. Moreover, even when cities do disclose information about their projects, the ethical implications and considerations are often unclear or insufficiently documented. This ambiguity makes it difficult to assess certain projects’ alignment with GOUAI’s ethical principles, resulting in the exclusion of some potential best practices.
By the same token, the overall data collection from primary sources was hindered by a low and sporadic response rate from city officials. Their limited availability and capacity to respond to data inquiries was a barrier to qualitative data collection. Even when city officials were willing to share information, they frequently encountered difficulties related to internal communication. This issue reflects the complexity of coordinating and disseminating information within city administrations.
3. The concept of “urban artificial intelligence”
Artificial intelligence is inherently connected with urban life. For one thing, urban spaces serve as tangible and prominent settings where AI is visibly present. Cities also function as testing grounds for new AI forms and are where some of its social and environmental impacts, in terms of both opportunities and risks, are most felt (Galceran-Vercher and Rodríguez Pérez, 2024). Furthermore, the functioning and development of AI require a variety of resources, many of which are closely tied to urban settings. In essence, cities can be described as microcosms through which AI observes and comprehends the wider world. More specifically, cities provide four crucial types of resources (Cugurullo et al., 2023b):
- Urban spaces: AI requires physical environments to act upon, and these are frequently urban. Examples include roads, buildings, parks, streets, etc.
- Access to activities: Urban settlements encapsulate a growing proportion and diversity of activities worldwide. Consequently, if an AI application is to partake in social interactions, participate in economic transactions, play a role in political processes or contribute to global environmental changes, it must interact with urban systems.
- Data for machine learning: Most AI systems presently enhance their intelligence through machine learning processes, which entail extensive data usage. Urban areas, characterised by dense populations, serve as significant hubs for diverse human activities, making them crucial producers of data. However, the importance of cities lies not only in the sheer volume of data they generate but also in the quality of the data. Urban spaces produce real-time data, which is highly detailed and particularly beneficial for machine learning applications.
- Infrastructures and facilities: Cities also offer other key facilities such as electrical grids, server farms, etc.
The concept of “urban artificial intelligence” seeks to underscore the relationship between algorithmic systems and urban contexts, encompassing the built environment, infrastructures, places, people and their social practices (Luusua et al., 2023). This can be defined as any system that employs data derived from the urban environment and processed through algorithms, yielding practical applications in the socio-spatial dynamics of the city (Popelka et al., 2023, p. 14). Broadly speaking, the urban incarnation of AI systems has taken four distinct forms (Cugurullo et al., 2023a): autonomous vehicles, urban robots, city brains and urban software agents.
Some important features distinguish urban artificial intelligence from other forms of artificial intelligence (Popelka et al., 2023). Firstly, the intricacy of the city: numerous interconnected sectors contribute to the functioning of urban areas. The Atlas of Urban AI categorises AI initiatives into seven such sectors: social services, governance and urban services, environment and resources, mobility, infrastructures and urban planning, economy and business, security and resilience. Similarly, many stakeholders partake in urban life: from the private sector to civil society organisations or grassroots communities. The second feature is the fact that urban AI operates within a specific policy context. Cities are political arenas where local governments possess the authority to make decisions that impact the lives of millions. Consequently, urban artificial intelligence applications wield a distinct political influence. Moreover, it is precisely the recognition of this political dimension that has led to the Atlasprioritising the compiling of cases where local governments are directly or indirectly involved, at least in the first stage of the research. The final feature of urban AI is its hybrid nature: urban AI cannot exist solely in the digital realm. Conversely, it necessitates the materiality and infrastructural components of physical urban systems.
As argued, the added value and distinctive nature of GOUAI’s Atlas of Urban AI lies in its emphasis on use cases with ethical considerations. This is important because the widespread deployment of AI systems in urban areas, connected to their impact on our everyday urban experiences and the process of city-making, is giving rise to numerous ethical concerns. Consequently, there is a growing need to examine the interconnections among artificial intelligence, the physicality of urban spaces, individual lives and the ethical and political aspects inherent to urban AI. In this respect, it is noteworthy that certain common uses of AI systems in urban environments have been categorised by the EU AI Act as either high-risk or limited-risk (Table 1). This implies that certain safeguards such as testing, documentation, transparency or notification duties must be implemented to mitigate potential harmful effects.
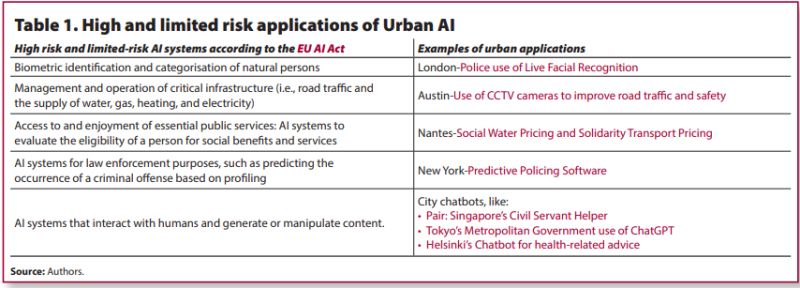
4. Trends overview
a. Europe and North America: strongholds of ethical AI?
Some 80% of the initiatives documented in the Atlas of Urban AI are located in North America and Europe, followed by 10% in Asia; 8% in Latin America and the Caribbean; and 1% in the MENA region. This uneven distribution (Figure 1) raises the question: is urban AI predominantly associated with the Global North? Indeed, a large part of the technology is developed by industries in this region, facilitating easier access for municipalities and positioning them as innovation hubs for its use. Furthermore, cities in Europe and North America typically possess more extensive resources, both technical and financial. They also have greater levels of political and fiscal autonomy to initiate, develop or regulate technological projects. These competences often fall under national responsibility in other regions of the world. As a matter of fact, many cities worldwide serve merely as a testbed for innovations pushed forward by the private sector, supranational organisations and other tiers of government. In such cases, the local government may not necessarily play an active role as a partner in these advancements.
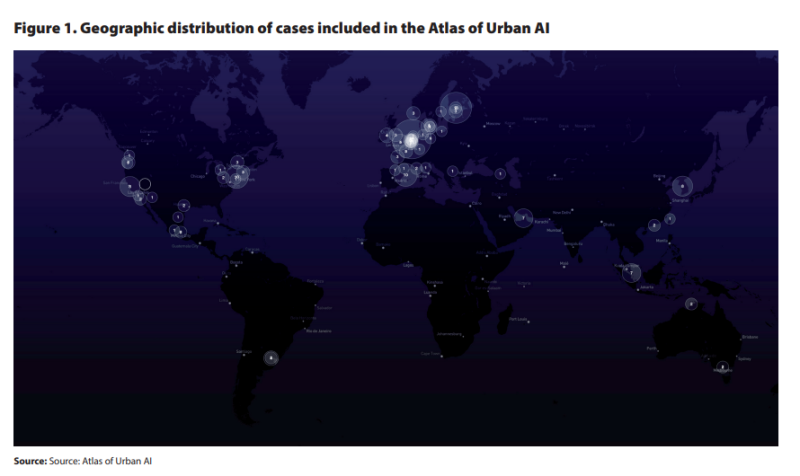
Nevertheless, misrepresentation is also exacerbated by other limitations (partly addressed in the methodological section of this report). Firstly, there are disparities in communication strategies, with European and American cities often employing more robust and outward-looking communication approaches. This visibility makes their projects more accessible to the general public compared to many of their counterparts. Secondly, geographical proximity plays a key role. This Atlas is a product of GOUAI, a joint project between CIDOB’s Global Cities Programme and the cities of Barcelona, Amsterdam and London, in the framework of the Cities Coalition for Digital Rights. Hence, communication channels are more easily established with cities already connected through these networks. However, geographical proximity also introduces other types of limitations such as language barriers and cultural disparities, as perspectives on ethical AI differ across regions. These realities hinder efforts to collect detailed information about urban AI initiatives in other parts of the world, impacting the comprehensiveness of the database and influencing the assessment of ethical AI practices worldwide. This underscores the importance of adopting a more inclusive and globally representative approach to data collection and ethical assessment in future research.
b. Top sectors for AI deployment: governance and urban services
As shown in Figure 2, an overwhelming majority of local governments use AI tools to improve governance and urban services. One illustrative example is the growing trend among cities worldwide to introduce city chatbots to assist residents and provide services (e.g. Dubai.AI). Moreover, several cities are increasingly leveraging AI tools to upgrade internal municipal management processes (e.g. The Hague), collect data to regulate public space (e.g. Taoyuan), manage and analyse urban data (e.g. Los Angeles), improve efficiency in urban services (e.g. Melbourne) or enhance law enforcement efforts (e.g. Seoul). Accounting for 66% of the total initiatives featured in the Atlas, the governance and urban services sector is the predominant category in urban AI, followed by mobility (24%), social services (22%) and environment and resources (22%).
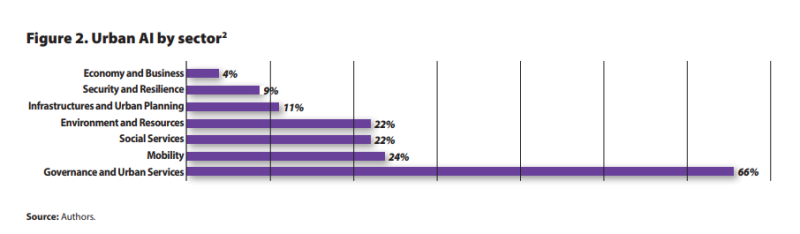
Meanwhile, the least represented sectors in the Atlas are infrastructures and urban planning (10%), security and resilience (9%), and economy and business (4%). In fact, only seven of the initiatives included in the Atlas are economy-oriented, featuring projects such as using AI to map commercial opportunities (e.g. Buenos Aires) or improve logistics for local industries (e.g. Rotterdam). These low figures may suggest a lack of interest on the part of municipalities in applying AI for productive purposes or economic gain, contrasting with the extensive use of AI for economic advancement globally. Conversely, security and resilience are high priorities for municipalities yet are underrepresented in the Atlas due to ethical considerations. For instance, the Atlas deliberately excludes controversial uses of facial recognition technologies, which are extensively employed by many cities worldwide. Instead, it includes responsible examples within this sector, ranging from the creation of biometric privacy laws targeted at businesses (e.g. New York), to the use of AI for early detection of fires (e.g. Hong Kong) or mapping perceived security (e.g. Stockholm).
c. Transparency and privacy protection: the golden ethical principles
Ethical principles that municipalities apply in urban AI implementation and development vary in their prevalence. Particularly noteworthy is the widespread adoption of the “transparency and openness” principle, observed in 67% of the initiatives included in the Atlas (see Figure 3). One plausible explanation for this prevalence is its perceived ease of application. This is mainly achieved by informing the public about the use of AI (i.e. when, how and with what purpose an AI system is being employed). This information is disseminated through open communication channels and platforms, including AI registers and inventories such as the ones established by the cities of Amsterdam, Nantes, Helsinki or San José. To a lesser extent, this principle can also be accomplished by using open-source and open data-initiatives whenever possible.
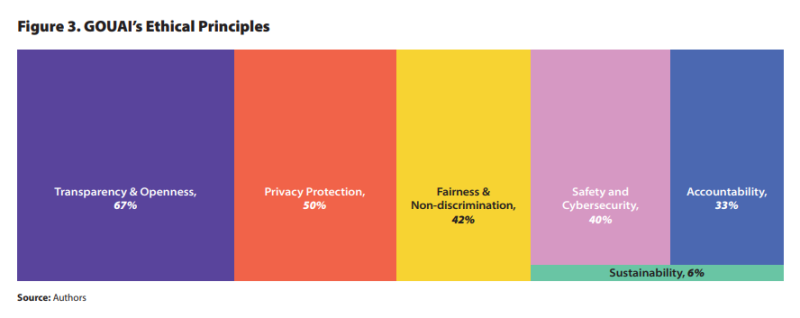
“Privacy protection”, identified in 50% of initiatives, stands as the second most upheld principle. Mainly accomplished through the anonymisation of data and, more recently, through the generation of synthetic data. Examples of this can be found broadly, from the anonymisation of licence plates and biometric information when employing image recognition tools (e.g. Greater Manchester’s Behaviour Analytics or Melbourne’s Smart Traffic Solution), to the use of lidar and radar technology to avoid capturing personal identifiable information in the first place (e.g. Amsterdam’s Roboat), or the creation of synthetic data frameworks (e.g. Dubai’s Synthetic Data Framework). In this context, the EU General Data Protection Regulation (GDPR) undoubtedly played a pivotal role in paving the way to an extensive culture of privacy-by-design.
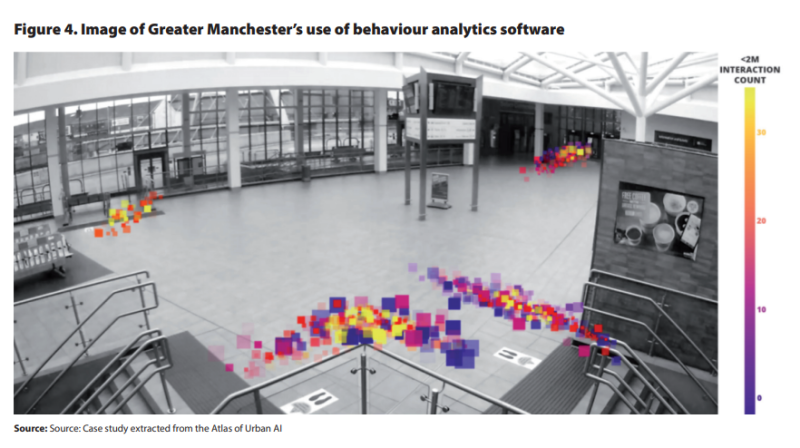
Conversely, the principles of “fairness and non-discrimination” (42%) and “safety and cybersecurity” (40%), along with “accountability” (33%), are applied unevenly, and are present in roughly 40% of initiatives. Common practices employed to observe these principles include conducting external audits, adopting a human-in-the-loop approach, and utilising technology to support decision-making rather than fully automating decisions. However, it should be noted that although external audits are a desirable measure to respect all three principles, private providers of AI systems are generally reluctant to disclose information on copyright protection grounds, resulting in the use of black-box technologies2 and the erosion of these principles.
When cross-referencing sectors and principles, we find there is indeed variability in the adoption of different ethical principles across sectors (see Figure 5). While transparency stands out as the most prevalent principle across all sectors, its significance is particularly pronounced in urban AI associated with the provision of social services. Considering that AI systems in this field are at times utilised to allocate social provisions, thus impacting the lives of the most vulnerable, it is imperative to establish safeguards to comprehend and, if necessary, challenge the decisions made. The principles of fairness and non-discrimination and accountability also carry considerable weight in social services for similar reasons.
A second observation is that some sectors need greater integration of various ethical principles more than others. Notably, initiatives related to mobility seem to incorporate fewer ethical provisions, primarily focusing on transparency and privacy protection considerations when included. A striking final observation is the insufficient attention given to the principle of sustainability in initiatives striving to enhance environmental conditions and resource allocation. This aspect will be further explored in the next section.
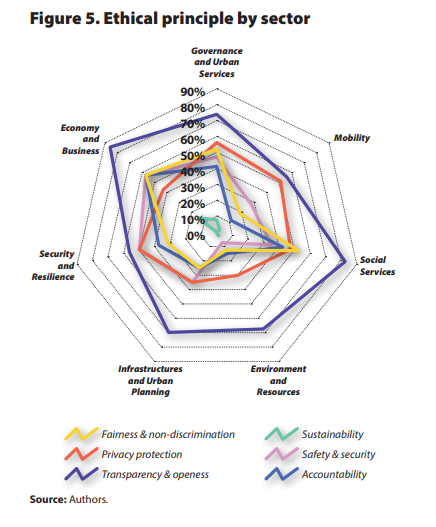
d. Sustainability: the elephant in the room
As argued, “sustainability” is by far the least commonly upheld principle, with only 6% of initiatives incorporating it. Instances where it is present mainly involve being named in strategies rather than being applied in projects themselves. The challenge lies in reconciling the dark side of AI – addressing the environmental and social impacts the use of this technology entails – with its use for achieving sustainable purposes. In essence, the sustainability of AI clashes with the use of AI for sustainability. From an environmental perspective, AI is energy- and resource- intensive by nature, generates considerable amounts of electronic waste and depends on the generally unsustainable extraction of rare metals and rare earths, incurring not only environmental, but also social and geopolitical costs. Strategies to achieve a greener AI include using sustainable energy to fuel green data centres and reuse their excess heat, training algorithms with minimal and small datasets rather than large ones, and considering ethical sourcing of materials.
Regarding social sustainability, AI and algorithmic systems at large have been unequivocally found to reproduce and reinforce existing societal biases. To effectively address this, cities need to embrace a digital rights perspective that ensures the respect of basic ethical principles. In this regard, initiatives such as the Cities Coalition for Digital Rights are key spaces for cities to implement operational frameworks to advance human rights in digital environments. On the other hand, the process of training AI itself also poses ethical concerns, as much of this “automated” training is in fact performed on many occasions by underpaid workers in dubious conditions in the Global South.
e. Exponential growth of urban AI: a technology here to stay
In terms of the timeframe, 64% of the cases compiled in the Atlas were initiated between 2017 and 2023, indicating a notable surge in the adoption of algorithmic systems by municipalities over the past five years (see Figure 6). Furthermore, 2021 was a turning point in the formulation of local strategies and policies to govern AI, aligning with a global trend of accelerated efforts to regulate AI.
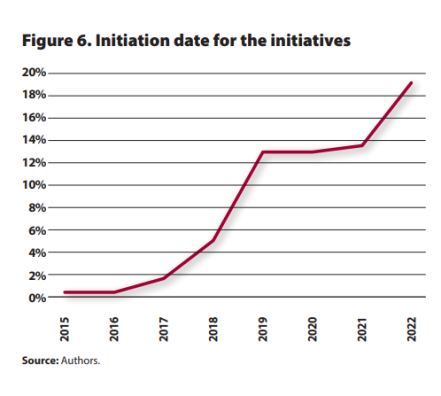
Regarding the maturity level of these initiatives (Figure 7), 66% are now fully implemented, while the rest are in the implementation or planning phase (17% in each case).3 Consequently, we can infer that many cities worldwide have moved beyond the pilot stage of this technology and are now actively employing it in a vast array of sectors in the execution of their day-to-day responsibilities. This growth is bound to increase exponentially in the years to come, sustained by the steady increase in global investments in AI development and the ever-growing popular interest in this technology.
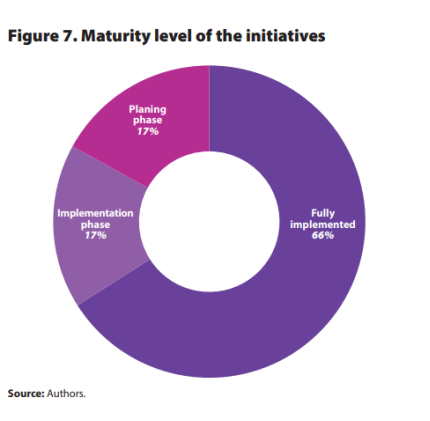
f. Urban AI: a collective multistakeholder endeavour
The majority of initiatives featured in the Atlasare the result of various forms of public-private partnerships, involving collaborations between cities, companies, knowledge institutions, and various international and supranational organisations, including the European Union and the United Nations. Moreover, there is a growing trend in city-to-city collaboration, often facilitated by international city networks like Eurocities. In this context, it is crucial to emphasise that most cities worldwide lack the financial resources and the technical capacity to develop their own AI solutions and projects, leading them to acquire this technology primarily through procurement channels.
This situation underscores the critical importance of alliances and collaborations, which have played a key role in encouraging cities of various sizes to adopt AI. Despite concerns about AI potentially perpetuating geographical inequalities seen in previous high-tech industries concentrated in leading tech hubs and “superstar” cities, our Atlas reveals that the AI revolution is not exclusive to large cities. Rather the opposite: 66% of the cities identified in our Atlas are small to medium-sized, encompassing populations ranging from 250,000 up to 1 million residents.
5. From AI adoption to AI governance: How are cities regulating AI?
The analysis of the types of urban AI initiatives included in the Atlas reveals that while many cities are actively innovating on AI use cases, few have put in place specific policies or comprehensive strategies to govern such adoption and ensure alignment with fundamental ethical principles. To quantify this, a significant 82% of the cases featured in the Atlas are specific AI-enabled urban services or solutions, with considerably lower percentages for policies (12%) and strategies (6%), as illustrated in Figure 8. To put it another way, a mere 11% of the cities documented in the Atlas have established specific AI strategies or action plans. Noteworthy examples among this select group of pioneering cities, include New York City’s AI Strategy (2021) and Action Plan (2023); Barcelona’s Municipal Strategy for promoting an ethical AI (2021); Agenda AI: Amsterdam Intelligence Agenda (2021); Buenos Aires’ AI Plan (2021); or Vienna‘s Artificial Intelligence Strategy (2019).
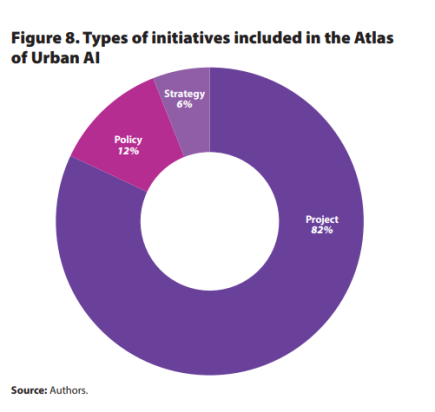
On the other hand, only 21% of them have put in place local policies and regulations designed to oversee AI in different ways. Instances of these policies encompass the introduction of procurement clauses, enactment of legislation to advocate for algorithmic transparency, creation of public algorithmic inventories and registries, establishment of councils or advisory commissions, and the formulation of guidelines. The table below provides a more extensive compilation of these policies and regulations, incorporating specific examples taken from GOUAI’s Atlas.
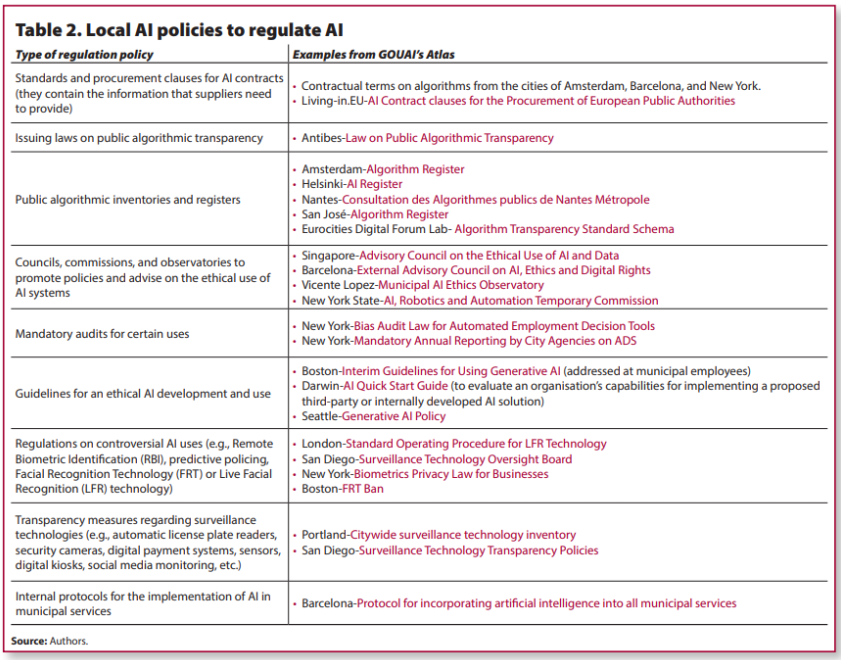
These findings indicate that there is at present an acute mismatch between the widespread adoption of algorithmic tools and the establishment of effective AI governance. This disparity is also evident in other spheres of technological governance worldwide. Arguably, the prevalent use of algorithmic tools without proper governance frameworks in place may indicate a general tendency towards a pragmatic and solution-oriented approach that municipalities (and other actors) have been embracing.
In this sense, the primary focus has until recently fallen on addressing immediate urban challenges by leveraging available technologies, including AI. In contrast, the governance of AI, characterised by a deliberate intent to comprehensively regulate the technology to minimise associated risks, has lagged behind. However, the recent popularisation of tools such as ChatGPT, Dall-e and others, has catapulted ethical concerns into the public discourse, prompting most governments and international actors to embark on a global race to regulate algorithms and AI. In light of this, local governments cannot afford to be excluded from this race. Therefore, we can only anticipate an increasing number of cities worldwide advocating for policies and regulations to govern AI. The main challenge ahead for the Global Observatory of Urban AI will be to take stock of all these efforts.
References
Cugurullo, F., et al., (2023a) “The rise of AI urbanism in post-smart cities: A critical commentary on urban artificial intelligence”. Urban Studies, Nov. 2023
Cugurullo, F., et al. (2023b) Artificial Intelligence and the city. Urbanistic perspectives on AI. London: Routledge
Galceran-Vercher, M. and Rodríguez-Perez, A. (2024) “The dark side of urban Artificial Intelligence: addressing the environmental and social impact of algorithms”. CIDOB Briefings, 55
Luusua, A., et al. (2023) “Understanding the emerging role of artificial intelligence in smart cities”, AI Soc., 38(3), pp. 1039-1044.
Popelka, S. et al. (2023) Urban AI Guide 2023. Urban AI
Notes:
1- Fairness and non-discrimination; transparency and openness; safety and cybersecurity; privacy protection; sustainability; and accountability
2- AI black boxes refer to AI systems with internal workings that are invisible to the user. You can feed them input and get output, but you cannot examine the logic that produced the output.
3- Some 28% of the initiatives lack available information regarding their maturity status and the year of initiation.